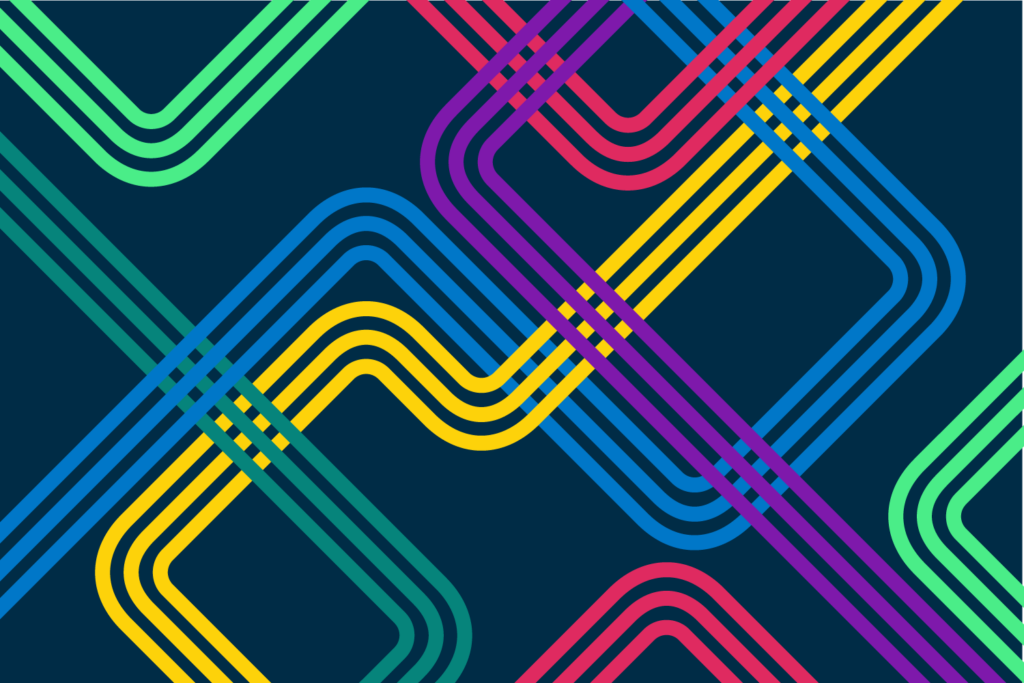
A Q&A with Eleanor Tursman and B Cavello of Aspen Digital, on the recently released AI Primers for Journalists
AI Primers for Journalists is the latest in a series of emerging tech primers researched, written, and published by Aspen Digital. While designed with journalists’ needs in mind, the three primers, which include AI 101, Intro to Generative AI, and Finding Experts in AI, are useful to anyone interested in unpacking the sometimes-confusing and jargon-heavy world of AI. We sat down with Siegel Research Fellow and Emerging Technology Researcher at Aspen Digital, Eleanor Tursman and Aspen Digital Director of Emerging Technologies, B Cavello, to discuss their approach to the work and what they hope to see come out of it in the future.
Where did the idea for the AI Primer for Journalists come from? What challenges were you seeking to address?
Eleanor Tursman: Aspen Digital has a history of briefing news media on emerging topics. Our executive director [Vivian Schiller] has a lot of experience in the space, and other team members are interested in journalism and information integrity.
When B joined, one of their big project ideas was to take difficult or complicated emerging tech topics and make them into digestible resources for different populations and audiences. We had already been working on AI more broadly when the surge in generative AI began last winter. All of a sudden there are all of these articles coming out about ChatGPT, Stability AI, Stable Diffusion, etc. that we found to be misleading on both what the tech was actually capable of and what might actually come once that tech was released into the wild. Between the generative AI hype cycle and the deep connections our team has to the journalist space, it felt like a good place to make a big impact by providing some kind of understandable resource for journalists.
B Cavello: The short answer is journalists asked us for this stuff. As Eleanor mentioned, we already have a longstanding program of working with journalists on a variety of different topics. And repeatedly reporters were being asked to cover stories on AI and realizing that it’s very difficult to find both trustworthy and understandable content on this topic. We talked to a bunch of different journalists as part of the process of developing these primers and the thing that we heard again and again is “there’s stuff out there and yet it’s not serving our needs.” And so part of what motivated this work was really trying to take user-centered view on what would be useful to journalists to speak to this issue.
What are the biggest problems with how we talk about AI in the popular press that you hope the primer can address? You mentioned trustworthiness and understandability as two. Are there others?
Eleanor Tursman: A lot of the material that’s out there is either too complicated or has too much undefined jargon. It also assumes a baseline of very technical knowledge.
Another issue is that many of the “experts” being interviewed are tech spokespeople, who knowingly or unknowingly feed back into the hype cycle rather than making the underlying technology understandable.
B Cavello: I think one of the most surprising challenges is that a lot of the issues that people are concerned about in AI – from basic automation to bias to job loss to the impact on major industries like automated vehicles – are things that may happen or we anticipate to happen, but haven’t necessarily happened yet at scale. Journalists are not speculative; they report on things that have already happened and may use those as a hook to pose questions. And so we had to be thoughtful about where we have documented examples right now, while also giving people enough hints where to look so that as this topic evolves and shows up in people’s lives, journalists are equipped to unpack those issues.
It sounds like you took a much different approach. How did you find out what questions journalists had about AI and what gaps needed to be filled by this primer?
B Cavello: It was a combination of things. We started by doing some one-on-one interviews with folks from different beats – from healthcare to real estate – where we asked basic questions like: Is AI showing up in your work? If so, how? What kind of questions do you have about these technologies? What do you wish you knew? How do you feel like your newsroom or your colleagues are handling these kinds of questions? What things do you use as source material right now? Where do you go to look for reliable information? Through these conversations, we began to understand where the gaps were.
At the same time, Eleanor had been compiling existing resources on AI, some of which targeted journalists, but many of which were more general intros. That work was taking place before generative AI took off.
And then, when everyone was suddenly talking about generative AI, we realized there was value in having some collective conversations as well. So in addition to doing targeted interviews, we did a series of salon dinners that brought together AI experts and journalists to talk openly about what they’re learning, what they’re seeing, and what questions they have. These group conversations allowed us to get diverse journalist perspectives in one space. Overall, we had representatives from 28 publications and 13 experts in AI participate over the two group events. Some were from big, international press orgs and some were from local city papers – both wondering how to talk about this stuff in their unique context. It was really helpful to hear kind of where people’s heads were at, what people, what journalists think is exciting or not exciting, as well as some of the challenges.
How did you recruit folks for the discovery and development?
Eleanor Tursman: It was a mixture of existing contacts and reaching into new networks. The initial interviews were specifically with folks who were NOT on the tech beat – people who had NEVER written a story about AI before. Several people we interviewed actually said to us “if I had AI on my desk as a story to potentially write about, I would not take it because it would be too intimidating.”
B Cavello: And yet AI is showing up everywhere – in the entertainment pages, in health, in labor. So we made a concerted effort to reach out to people from a variety of different backgrounds. We also tried to capture a range of different levels, from senior editors to people coming out of J[ournalism] school, in an effort to understand what training or familiarity they had across different contexts.
Our executive director also has a deep network in news media. Our whole team – not just Eleanor and myself – has done a lot of work with journalists on how to cover a variety of topics, like cybersecurity, election reporting, and climate. As a result, we were able to bring in folks who were already kind of familiar with our work as well as try to extend our network a little bit and target non-tech beat journalists.
In interviews with journalists, [we learned that] the number one place that journalists go to get information on a new topic is other journalists. But you can only fit so many journalists in a room. And so we wanted to create something that was scalable and shareable, and something that didn’t rely on people having been to a conference, but that could be passed around, like a link to a page with useful resources.
A piece of really constructive feedback that we got was from a very senior journalist who challenged us to check how diverse the different sources of the articles that we link to are, both in terms of publication and authorship. Are we representing women journalists? Are we representing people of color? It was like a really good reminder to us to do that work. And so we make a concerted effort to represent a diversity of sources and viewpoints.
That said, some sections, especially the section on intellectual property, are not as diverse as we would like So if anyone has great resources on intellectual property and generative AI that we should be pointing to, we would love to diversify that section especially!
How is the primer different from other AI guides/information you have seen? What criteria guided you as you endeavored to tackle the complexity of AI in a way that was clear and concise enough to be useful to journalists?
Eleanor Tursman: There’s precedent of guidelines for reporting on complicated or difficult topics, like suicide, substance use disorder, or disinformation. However, there’s not a ton of consensus about how to report on AI.
We looked at tons of AI primers and briefs, including a few for journalists. A lot of what exists still has embedded jargon or uses language that is misleading. To make matters worse, a lot of the jargon are also words we use everyday. For example, a lot of the primers will use the phrase “trained the AI” without saying what “trained” actually means. We know what the dictionary definition of “trained” is, but in this context, it’s a catch-all that might describe many different technical approaches. Without unpacking what “trained an AI” means in an accessible way, it loses its meaning. We spent a lot of time thinking about the definitions we included in the primer.
Another issue is anthropomorphizing algorithms. Using terms like “the machine did X” or “the machine thinks Y.” It makes sense to a layperson, but it hides the human intention that went into producing the tech. AI doesn’t “make” decisions. Both the person using the tool and the people who created it are the key actors. By removing the human component, we remove accountability.
B Cavello: Exactly. Like we say “an algorithm fired 500 workers.” Well, it wasn’t the algorithm that fired those workers…it was the management that fired those workers, and they may or may not have used an algorithm in the process. But by saying it was the algorithm, they’re hiding behind a non-human entity to make a decision.
Eleanor Tursman: A third distinguishing factor is that many primers are focused on general audiences and not journalists specifically. Notably, they don’t list places where you can find experts. There are hundreds of machine learning and computer science conferences out there, but they are extremely dense and not very press friendly. There are also a lot of tech expos where you’re talking to a spokesperson, so it’s hard to cut through to bigger picture questions. And it also matters which type of role the interviewee holds; developers, designers, researchers, policy people, product people, C-suite executives – they all bring a different point of view. We wanted to provide a set of conferences where science or technology or policy experts gather so journalists had some direction on how to find experts. That was something we hadn’t seen in existing primers. We wanted to help journalists break out of the cycle where they’ve read one expert interview and think “I’m going to interview that expert too,” and then you end up with the same five men being interviewed.
B Cavello: We got some tough love feedback from people keeping it real with us. They told us what was useful, not useful, too long, etc. We really tried to listen to the needs that journalists express. For example, they told us to put definitions in line so they can copy/paste it from where it is in the sentence instead of having to refer to an appendix at the end with a bunch of definitions. Or signposting where information is so it can be easily found. And then, as Eleanor said, it’s incredibly important that they know where to find trustworthy sources of information and recognize that there are many different points of view in an emerging topic like AI. So giving them multiple places to look, accompanied by a bit of context-setting.
Taking a step back, what do you wish generally more people understood about AI?
Eleanor Tursman: I wish more people realized that AI is not the technology of science fiction, and that an algorithm does not need to be “smart” to cause harm . Very, very “not smart” algorithms are hurting people at scale already. For example, automated systems are used to deny medical claims for people on Medicare Advantage, rather than a human denying service. Automation can make an existing bad system work badly at scale. And it’s frustrating to see so much focus on far off things like the “intelligence” question, rather than how technology can both help and hurt people right now, even technology that’s not “smart.”
What’s the difference between “smart” and “not smart”? I think of “not smart” tech as having very defined rules given to it by humans, whereas “smart” tech figures out the rules as it goes along.
Take the example of personalizing a lesson plan. A classic or “not smart” piece of tech might use just an “if-then” statement that says, “if the student gets above 80% on this quiz, then pass them to the next level, otherwise fail them and make them take it again.” [Humans] have defined all of the steps ahead of time. On the other hand, “smart” tech makes up rules as it goes through data science processes. If the student were to get a wrong answer about the solar system, you could instruct a piece of “smart tech” to give the student another question about the solar system, but what that question is would be based on huge amounts of test data from other students who have also failed that question.
If there’s a human expert defining all of the rules necessary for how a system operates – that’s a normal or “not smart” algorithm. If you have the algorithm generate the rules itself, that’s closer to something I’d call “smart.”
B Cavello: A lot of the current harms and potential risks are associated with the automation of processes that are already harmful. It’s not a question of technology doing something mysterious and unknowable, but rather that we are using technology to speed up and scale up already broken processes.
Another thing that I wish people understood is that these technologies are built by and used by humans. And I’m not just talking about the data sets, but also the assumptions about what “good” looks like, what our goals are, and how we define “success.” We should be aware of who’s making those decisions and how the people involved in those processes may lead us to build systems that are used in really harmful ways.
Lastly, I really wish people understood that AI automations are already everywhere in incredibly mundane ways, whether it be the autofocus in your camera, the spell check on your computer, or auto complete in your text messages. And so when people ask ‘what does all of this have to do with me?’ I think that understanding that these technologies are being increasingly built into all sorts of parts of our lives is a really good reason to care about these issues and to care about the way that the public talks about and learns about these issues.
What’s the impact you hope to see? What does success look like and how will you know when you start to see it?
B Cavello: There are a couple of leading indicators that we’re on the lookout for. First, while we have just begun our socialization process of sharing this around with people, I’m really excited for the day when it’s getting shared back to us. Like when we ask people “where do you go for information?” and they include our primers in the list of resources – that is gonna be a huge win.
A second indicator is if we see the language of the primers actually turning up in reporting on these issues. As Eleanor mentioned, we’re making a push to not personify the technology, which I know isn’t gonna be as attractive to some people. But if we succeed, hopefully we’ll have broken it down enough that we will start to see less of that language out in the world.
A third indicator is what we hear back from journalists. There’s a lot we packed into the primers, but also a lot left unsaid. I’m excited for the moment where people ask us questions and want to have a public conversation about the primers. For example, I’d love to see a Twitter thread of like, “how dare Aspen Digital leave out this really important thing?” Honestly, it would be great to have more of a meta conversation around the way we talk about technology generally.
And finally, I’d love to see [the primers] inspiring other people to create versions of these that are really user-centered targeted resources for journalists – or for other populations as well. But we’re not the only players in the space, and we certainly welcome partnerships with other organizations to find ways to share this work, to learn from the meta takeaways of the work, and perhaps even build future primers in partnership with us or on their own.